Machine Learning (ML) stands at the forefront of technological innovation, transforming the way computers process information and make decisions. This groundbreaking field of artificial intelligence has permeated diverse industries, from healthcare to finance, revolutionizing the landscape of data analysis and pattern recognition.
In this comprehensive exploration, we delve into the intricacies of machine learning, its underlying principles, applications, and the transformative impact it has on our rapidly evolving digital world.
Defining Machine Learning:
At its core, Machine Learning is a subfield of artificial intelligence that empowers computers to learn and improve their performance without being explicitly programmed. Rather than relying on explicit instructions, ML algorithms leverage data to recognize patterns, make predictions, and adapt to evolving scenarios. This ability to learn from experience positions ML as a versatile tool for tackling complex problems that traditional programming approaches may struggle to address.
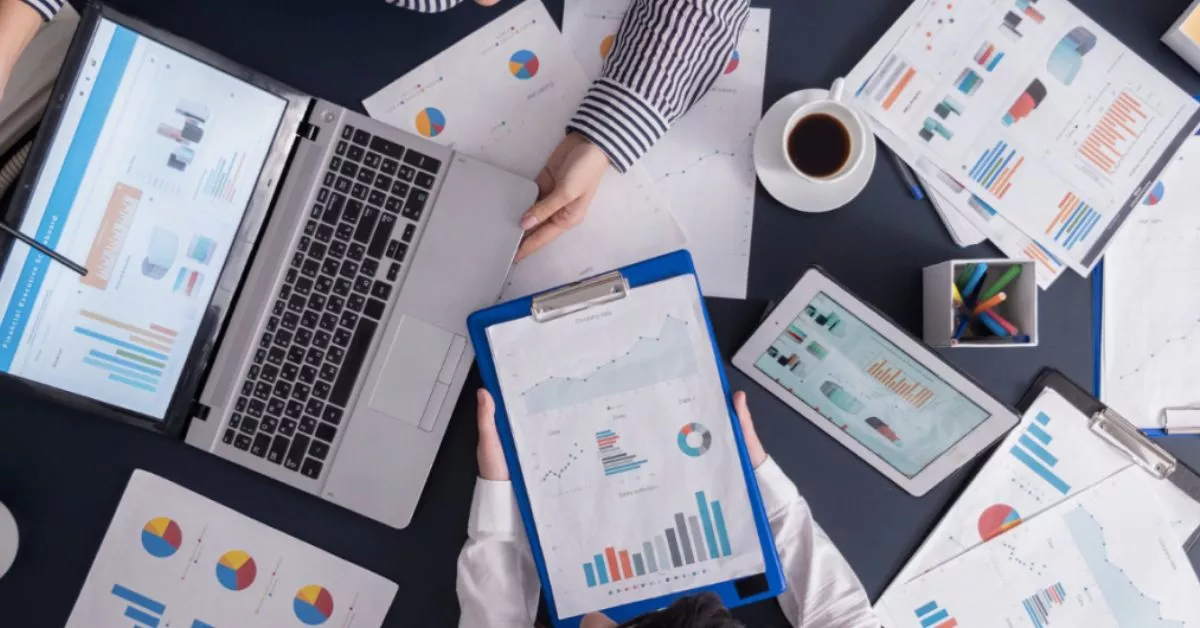
The Pillars of Machine Learning:
a. Supervised Learning: The algorithm is trained on a labeled dataset, where the input data is paired with corresponding output labels. The model learns to map inputs to outputs, making predictions on new, unseen data. This approach is prevalent in tasks like image recognition, speech recognition, and regression analysis.
b. Unsupervised Learning: Unsupervised learning involves working with unlabeled data, allowing the algorithm to identify patterns and relationships without explicit guidance. Clustering and dimensionality reduction are common unsupervised learning applications, aiding in tasks such as customer segmentation or anomaly detection.
c. Reinforcement Learning: Reinforcement learning involves training an agent to make decisions within an environment by receiving feedback in the form of rewards or penalties. This approach is prominent in applications like game playing, robotic control, and autonomous systems, where the algorithm learns through trial and error.
The Role of Neural Networks:
Central to many machine learning advancements are neural networks, computational models inspired by the human brain’s interconnected neurons. Deep Learning, a subset of ML, employs deep neural networks with multiple layers (deep layers) to extract intricate features and representations from data. Convolutional Neural Networks (CNNs) excel in image recognition, while Recurrent Neural Networks (RNNs) prove effective in sequential data tasks like natural language processing.
Applications Across Industries:
a. Healthcare: Machine Learning has revolutionized healthcare, enabling predictive analytics for disease diagnosis and treatment planning. ML algorithms analyze medical records, imaging data, and genomic information, contributing to personalized medicine and early detection.
b. Finance: In the financial sector, ML is employed for fraud detection, risk assessment, algorithmic trading, and customer service. Predictive models analyze market trends, optimizing investment strategies and enhancing decision-making processes.
c. Manufacturing and Industry 4.0: ML plays a pivotal role in Industry 4.0, where smart factories leverage predictive maintenance, quality control, and supply chain optimization. ML algorithms analyze sensor data to anticipate equipment failures, reducing downtime and improving overall efficiency.
d. E-commerce and Recommendation Systems: E-commerce platforms harness ML for recommendation systems, enhancing user experience by providing personalized product suggestions based on browsing history and preferences. This not only improves customer satisfaction but also drives sales.
e. Natural Language Processing (NLP): ML is the driving force behind Natural Language Processing applications, enabling machines to understand, interpret, and generate human language. Chatbots, language translation, and sentiment analysis are among the many applications in this domain.
Challenges and Considerations:
a. Data Quality and Bias: ML algorithms heavily rely on the quality of training data, and biased datasets can result in skewed predictions. Ensuring data quality and addressing biases is crucial for ethical and accurate machine learning outcomes.
b. Interpretability and Explainability: As ML models become more complex, their decision-making processes may seem like “black boxes.” Ensuring the interpretability and explainability of ML models is essential for building trust and understanding their implications.
c. Overfitting and Generalization: Overfitting occurs when a model becomes too tailored to the training data, potentially performing poorly on new, unseen data. Striking a balance between complexity and generalization is a perpetual challenge in ML.
d. Security and Adversarial Attacks: ML models are susceptible to adversarial attacks, where malicious actors manipulate input data to deceive the system. Enhancing the security of ML algorithms and developing defenses against adversarial attacks is an ongoing concern.
Future Trends and Innovations:
a. Explainable AI: The demand for explainable AI continues to grow, prompting research into creating models that provide transparent insights into their decision-making processes. Explainable AI is crucial, particularly in sensitive applications like healthcare and finance.
b. Federated Learning: Federated Learning decentralizes the training process, allowing models to be trained across multiple devices without sharing raw data. This approach maintains privacy and addresses concerns related to centralized data storage.
c. AI Ethics and Responsible AI: As AI becomes more pervasive, ethical considerations gain prominence. The development of AI ethics frameworks and responsible AI practices is essential to ensure fair, unbiased, and accountable machine learning applications.
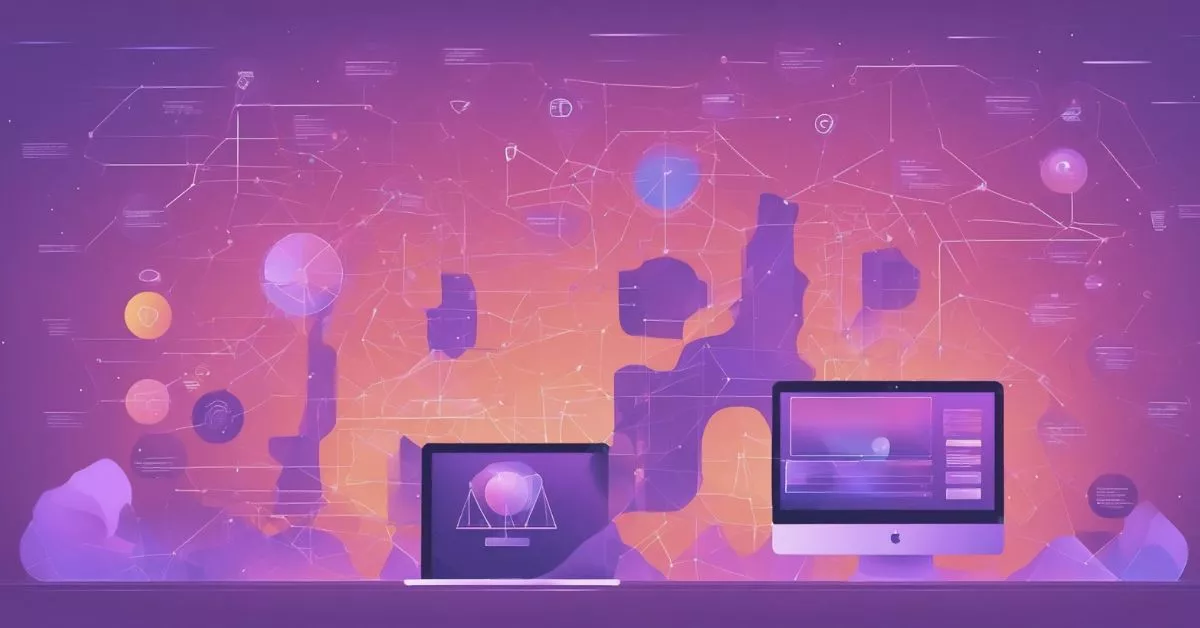
Conclusion: Shaping the Future with Intelligent Machines:
Machine Learning stands as a cornerstone of the technological revolution, empowering intelligent machines to learn, adapt, and evolve. Its applications span diverse industries, promising advancements in healthcare, finance, manufacturing, and beyond. However, as we navigate the evolving landscape of ML, addressing challenges related to data quality, interpretability, and ethics is crucial for ensuring a responsible and impactful integration of this transformative technology into our daily lives.